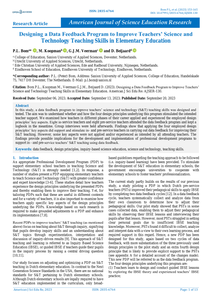
Designing a data feedback program to improve teachers’ science and technology teaching skills in elementary education
Published at:
Published at:
Published at:
Published at:
Published at:
Published at:
Published at:
Published at:
Published at:
Published at:
Lecturer and Researcher in Sociology, Methodology and Data Science
Lector Industriële Digital Twins
Onderzoeker
Start:
End:
Start:
End:
Start:
End:
Start:
End:
Start:
End:
Start:
End:
Start:
End:
Start:
End:
Start:
End: