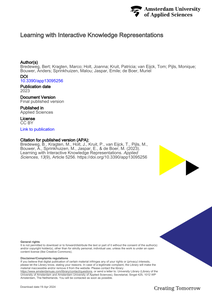
Learning with Interactive Knowledge Representations
Gepubliceerd op:
Gepubliceerd op:
Gepubliceerd op:
Gepubliceerd op:
Gepubliceerd op:
Gepubliceerd op:
Gepubliceerd op:
Gepubliceerd op:
Gepubliceerd op:
Gepubliceerd op:
Lecturer and Researcher in Sociology, Methodology and Data Science
Teacher Researcher
Start:
Eind:
Start:
Eind:
Start:
Eind:
Start:
Eind:
Start:
Eind: